Welcome
I am Professor of Statistics within the Department of Mathematical Sciences at the University of Bath. On these pages you will find information about my research and professional activities.
I am an active member of the SAMBa Centre for Doctoral Training.
Research
My research interests include:
- Multiscale methods in statistics, in particular wavelet lifting schemes
- Applications of wavelets in time series analysis
- Applications of wavelets in image processing, particularly biomedical imaging
- Variance stabilization through variable transformation
- Efficient statistical inference and Approximate Bayesian Computation
- Statistical network analyisis (processes on networks, time-varying networks)
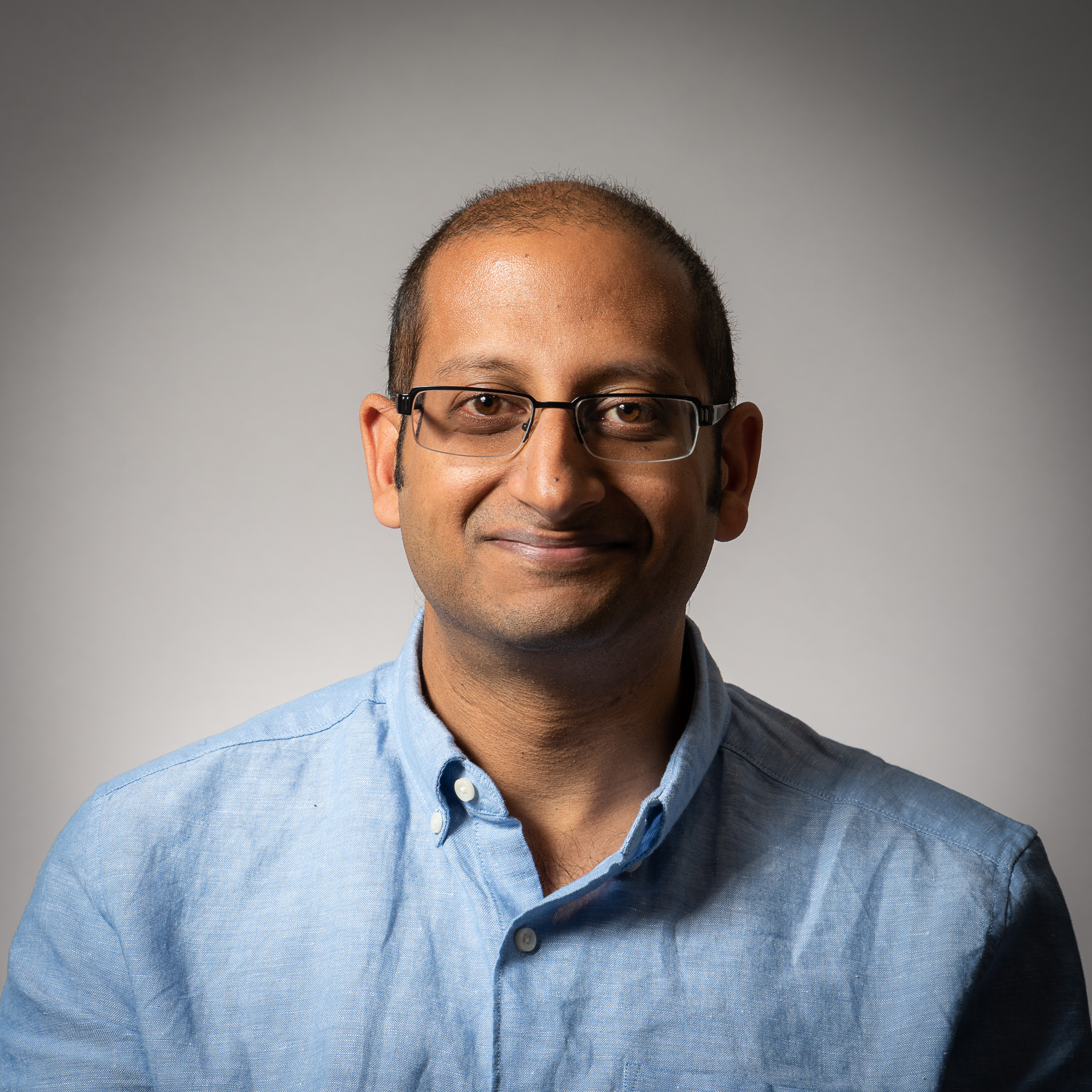
Matthew Nunes
Professor of Statistics
Department of Mathematical Sciences
University of Bath
Bath
BA2 7AY
Email: m.a.nunes (at) bath.ac.uk
You can find more about my research and publications on my Bath Research Portal page or see the publications page.
If you are interested in studying for a PhD with me or collaboration, then please contact at the email address on the right.
Example PhD topics include:
1. Tools for nonstationary time series. Many time series have a second order structure (variance properties) which are not constant over time; examples include volatility time series (log-returns), earthquake signals, ECG. The project would develop new tools for analyzing such time series, for example determining whether data has been sampled enough (absence of aliasing), real-time processing, testing suitability of models etc. For example, this could be due to faulty measurement devices or infrequent event data from environmental processes. Many models do not properly take this structure into account, which can lead to inaccurate modelling and conclusions being drawn. This project would focus on developing new models for such data.
2. Modelling irregularly spaced time series. Many time series have a natural irregular sampling structure, or feature missingness. For example, this could be due to faulty measurement devices or infrequent event data from environmental processes. Many models do not properly take this structure into account, which can lead to inaccurate modelling and conclusions being drawn. This project would focus on developing new models for such data.
3. Complex-valued signal processing. Whilst much data is real-valued, there are many situations where data can be viewed as complex-valued, for example when there is a directional and magnitude component. In addition, it has been shown that complex-valued tools can benefit analysis of real-valued signals. This project will investigate a number of methodological developments to unlock hidden information in real- and complex-valued data using complex-valued modelling approaches.
4. Long memory. It has been well established that many time series, from physiological data to climatic series exhibit long memory, i.e. a dependence structure which lasts over long periods. Accurate estimation of measures of persistence can be useful in climate modelling, however, traditional methods often suffer from bias. We would work on new methods of efficient estimation of these dependence measures in a range of time series and image settings.
5. Network data. There has been an explosion of data on networks, from epidemiological processes, to social media. However, not much work has been done linking the dynamics of the network itself together with the process observed on the network. The combine elements from time series and network analysis for computationally efficient inference methods for dynamic processes.
If you are interested in studying for a PhD with me or collaboration, then please contact at the email address on the right.
Example PhD topics include:
1. Tools for nonstationary time series. Many time series have a second order structure (variance properties) which are not constant over time; examples include volatility time series (log-returns), earthquake signals, ECG. The project would develop new tools for analyzing such time series, for example determining whether data has been sampled enough (absence of aliasing), real-time processing, testing suitability of models etc. For example, this could be due to faulty measurement devices or infrequent event data from environmental processes. Many models do not properly take this structure into account, which can lead to inaccurate modelling and conclusions being drawn. This project would focus on developing new models for such data.
2. Modelling irregularly spaced time series. Many time series have a natural irregular sampling structure, or feature missingness. For example, this could be due to faulty measurement devices or infrequent event data from environmental processes. Many models do not properly take this structure into account, which can lead to inaccurate modelling and conclusions being drawn. This project would focus on developing new models for such data.
3. Complex-valued signal processing. Whilst much data is real-valued, there are many situations where data can be viewed as complex-valued, for example when there is a directional and magnitude component. In addition, it has been shown that complex-valued tools can benefit analysis of real-valued signals. This project will investigate a number of methodological developments to unlock hidden information in real- and complex-valued data using complex-valued modelling approaches.
4. Long memory. It has been well established that many time series, from physiological data to climatic series exhibit long memory, i.e. a dependence structure which lasts over long periods. Accurate estimation of measures of persistence can be useful in climate modelling, however, traditional methods often suffer from bias. We would work on new methods of efficient estimation of these dependence measures in a range of time series and image settings.
5. Network data. There has been an explosion of data on networks, from epidemiological processes, to social media. However, not much work has been done linking the dynamics of the network itself together with the process observed on the network. The combine elements from time series and network analysis for computationally efficient inference methods for dynamic processes.
Other links
Finally, you can find out more about me here.